Manifold Learning for Super Resolution
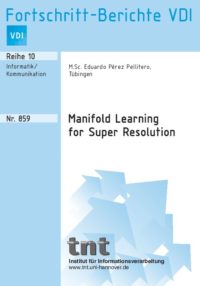
Erscheinungsdatum: 10.04.2018
Reihe: 10
Band Nummer: 859
Autor: M.Sc. Eduardo Pérez Pellitero
Ort: Tübingen
ISBN: 978-3-18-385910-8
ISSN: 0178-9627
Erscheinungsjahr: 2018
Anzahl Seiten: 128
Anzahl Abbildungen: 47
Anzahl Tabellen: 19
Produktart: Buch (paperback, DINA5)
Produktbeschreibung
The development pace of high-resolution displays has been so fast in the recent years that many images acquired with low-end capture devices are already outdated or will be shortly in time. Super Resolution is central to match the resolution of the already existing image content to that of current and future high resolution displays and applications. This dissertation is focused on learning how to upscale images from the statistics of natural images. We build on a sparsity model that uses learned coupled low- and high-resolution dictionaries in order to upscale images, and move towards a more efficient L2 regularization scheme. Instead of using a patch-todictionary decomposition, we propose a fully collaborative neighbor embedding approach. We study the positive impact of antipodally invariant metrics for linear regression frameworks, and extend them by also taking into consideration the dihedral group of transforms (i.e. rotations and reflections), as a group of symmetries within the highdimensional manifold. Our experimental section confirms that such approach greatly improves the PSNR performance and also obtains smaller dictionary sizes.
Keywords: Super Resolution, Manifold Learning, Machine Learning, Computer Vision Image Processing, Inverse Problems, Upscaling, Super Resolution, Manifold Learning, Machine Learning, Computer Vision Image Processing, Inverse Problems, Upscaling
* Der VDI-Mitgliedsrabatt gilt nur für Privatpersonen